Length: 2 Days
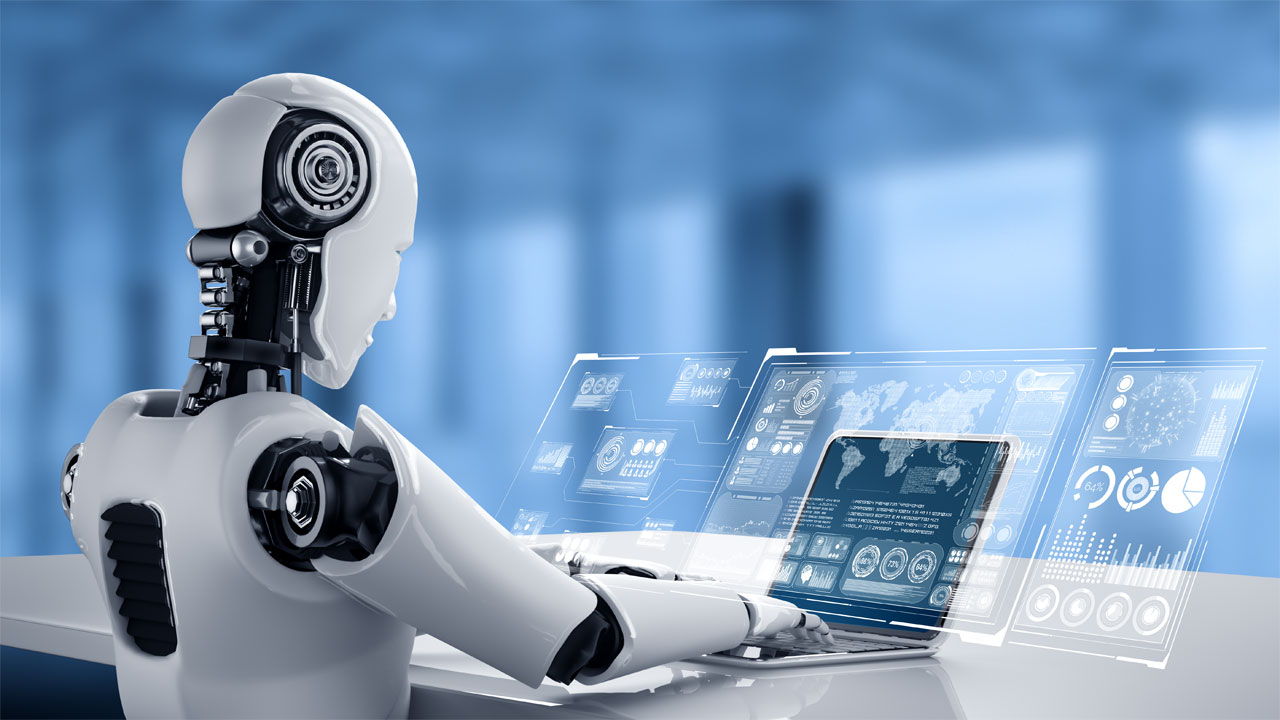
The Master of AI Security™ (MAIS™) Certification Course by Tonex is a comprehensive program designed to equip professionals with the knowledge and skills to safeguard artificial intelligence systems. This advanced course covers key aspects of AI security, addressing emerging threats and vulnerabilities in AI environments.
Tonex’s Master of AI Security™ certification course is a comprehensive program for cybersecurity professionals and AI enthusiasts, covering risk assessment, security measures, detection, response, and ethical considerations in AI. It equips participants with hands-on exercises and case studies to safeguard AI systems.
Learning Objectives:
- Understand the fundamentals of AI and its security implications.
- Learn techniques to assess and mitigate AI-specific risks.
- Master the implementation of security measures in AI systems.
- Gain expertise in detecting and responding to AI-related cyber threats.
- Explore ethical considerations and compliance in AI security.
- Acquire hands-on experience through practical exercises and case studies.
Audience: This course is ideal for cybersecurity professionals, AI developers, IT managers, and anyone involved in the deployment and management of AI systems. It is tailored for individuals seeking to enhance their expertise in securing artificial intelligence technologies.
Pre-requisite: None
Course Outline:
Module 1: Introduction to AI Security
- Overview of AI Security
- Evolution of AI Threat Landscape
- Risks and Challenges in AI Environments
- Importance of AI Security in Modern Context
- Key Terminologies in AI Security
- Future Trends and Emerging Technologies in AI Security
Module 2: Risk Assessment in AI
- Identifying Threat Vectors in AI
- Vulnerabilities Specific to AI Systems
- Risk Evaluation Methodologies for AI
- Impact Analysis of AI-Related Risks
- Quantifying and Prioritizing AI Security Risks
- Incorporating AI Security into Enterprise Risk Management
Module 3: Implementing AI Security Measures
- Securing Machine Learning Algorithms
- Best Practices for Securing AI Models
- Data Security Strategies for AI Datasets
- Encryption Techniques for AI Systems
- Authentication and Authorization in AI Environments
- Securing AI Deployment Pipelines
Module 4: Detection and Response in AI Security
- Strategies for Detecting Anomalous AI Behavior
- Monitoring and Logging in AI Systems
- Incident Response Protocols for AI Threats
- AI-specific Threat Intelligence
- Adaptive Security Measures for AI
- Continuous Monitoring in AI Environments
Module 5: Ethical Considerations and Compliance
- Ethical Guidelines in AI Security
- Responsible AI Practices
- Regulatory Landscape for AI Security
- Compliance with AI-related Standards
- Privacy and Legal Considerations in AI Security
- Transparency and Accountability in AI
Module 6: Hands-on Practical Exercises
- Real-world Simulations in AI Security
- Case Studies on AI Security Incidents
- Application of Security Measures in AI Scenarios
- Practical Implementation of AI Security Protocols
- Hands-on Experience with AI Security Tools
- Collaborative Problem Solving in AI Security Exercises
Course Delivery:
The course is delivered through a combination of lectures, interactive discussions, hands-on workshops, and project-based learning, facilitated by experts in the field of AI security. Participants will have access to online resources, including readings, case studies, and tools for practical exercises.
Assessment and Certification:
Participants will be assessed through quizzes, assignments, and a capstone project. Upon successful completion of the course, participants will receive a certificate in Master of AI Security.
Exam Domains:
- Foundations of AI Security:
- Understanding of basic concepts in artificial intelligence and machine learning.
- Knowledge of common security threats and vulnerabilities in AI systems.
- Familiarity with encryption techniques and secure data handling in AI applications.
- Adversarial Machine Learning:
- Recognition of adversarial attacks and techniques to mitigate them.
- Understanding of robust machine learning models and their implementation.
- Knowledge of techniques such as adversarial training and model distillation.
- Secure AI Model Development:
- Proficiency in developing AI models with security considerations.
- Understanding of secure coding practices for AI algorithms.
- Knowledge of model explainability and interpretability for security auditing.
- AI Ethics and Privacy:
- Awareness of ethical considerations in AI development and deployment.
- Understanding of privacy-preserving techniques for AI systems.
- Knowledge of regulatory frameworks and compliance requirements for AI security and privacy.
- Secure Deployment and Operations:
- Competence in securely deploying AI models in production environments.
- Familiarity with secure DevOps practices for AI systems.
- Understanding of continuous monitoring and incident response for AI security.
Question Types:
- Multiple Choice: Assessing conceptual understanding and factual knowledge.
- Scenario-based Questions: Evaluating practical application of AI security principles in real-world situations.
- Problem Solving: Testing the ability to identify and address security vulnerabilities in AI systems.
- Case Studies: Analyzing and providing recommendations for AI security and privacy challenges.
Passing Criteria:
- Minimum Passing Score: 70%
- Comprehensive Understanding: Demonstrating proficiency across all exam domains.
- Practical Application: Showing the ability to apply AI security principles to real-world scenarios.
- Critical Thinking: Exhibiting problem-solving skills and analytical thinking in addressing AI security challenges.